Introduction:
Artificial Intelligence (AI) has become increasingly integrated into various aspects of our lives, from recommendation systems to decision-making processes. However, as AI systems become more prevalent, it’s crucial to address the ethical considerations surrounding their development and deployment. One of the most pressing issues is bias and fairness in AI algorithms. In this blog post, we’ll explore the importance of ethical AI, the challenges of bias, and strategies for ensuring fairness in AI systems.
Understanding Ethical AI:
- Define what ethical AI means and why it’s important.
- Discuss the potential risks of unethical AI, such as perpetuating societal biases, violating privacy, and impacting human rights.
- Highlight the need for transparency, accountability, and responsible AI development practices.
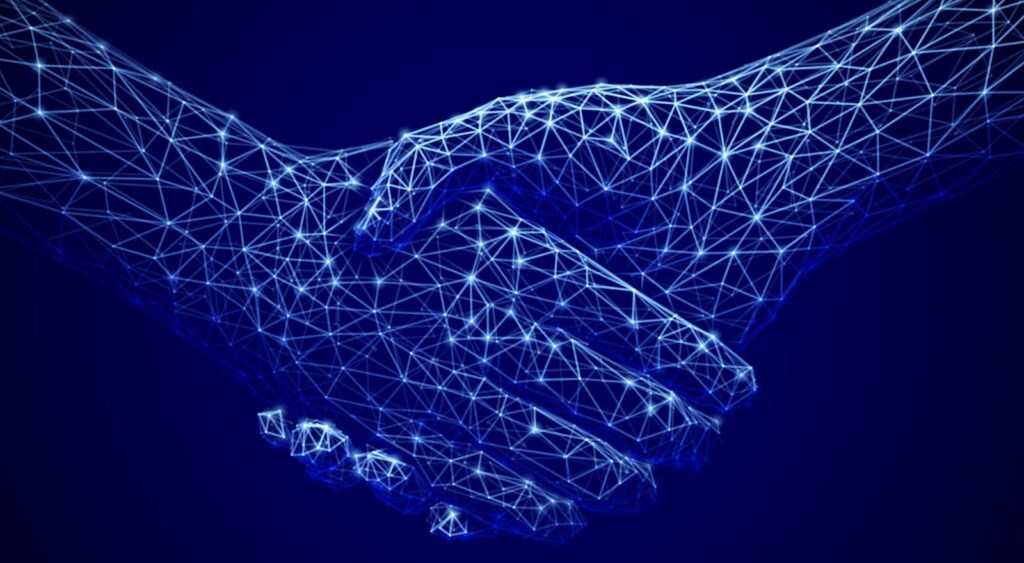
The Challenge of Bias in AI:
- Explore the various types of biases that can manifest in AI algorithms, including data bias, algorithmic bias, and societal bias.
- Provide examples of real-world scenarios where bias in AI systems has resulted in unfair outcomes or discrimination.
- Discuss the root causes of bias, such as skewed training data, algorithmic limitations, and human biases encoded in AI models.
Algorithmic Transparency and Explainability:
- Explore the importance of transparency and explainability in AI systems to facilitate understanding and accountability.
- Discuss techniques for making AI algorithms more interpretable, such as model documentation, feature importance analysis, and post-hoc explanations.
- Address the challenges of balancing transparency with proprietary concerns and trade secrets in commercial AI applications.
Diversity and Inclusivity in AI Development:
- Advocate for diverse representation and inclusivity in AI development teams to mitigate bias and ensure that AI systems reflect the needs and perspectives of diverse populations.
- Discuss the importance of diverse training data that accurately represents the full range of human experiences and identities.
- Highlight initiatives aimed at promoting diversity in AI, such as mentorship programs, scholarships, and community outreach efforts.
Strategies for Ensuring Fairness:
- Outline techniques for detecting and mitigating bias in AI algorithms, such as bias audits, fairness-aware machine learning, and diverse training data.
- Highlight the importance of interdisciplinary collaboration between data scientists, ethicists, policymakers, and community stakeholders in addressing bias.
- Discuss the role of regulatory frameworks and guidelines, such as the EU’s General Data Protection Regulation (GDPR) and the IEEE’s Ethically Aligned Design, in promoting fairness and accountability in AI development.
Case Studies and Best Practices:
- Showcase real-world examples of organizations or research initiatives that have successfully implemented ethical AI practices to mitigate bias and promote fairness.
- Highlight best practices for designing and deploying AI systems that prioritize fairness, diversity, and inclusivity.
- Discuss the ethical dilemmas and trade-offs involved in balancing fairness with other objectives, such as accuracy and efficiency.
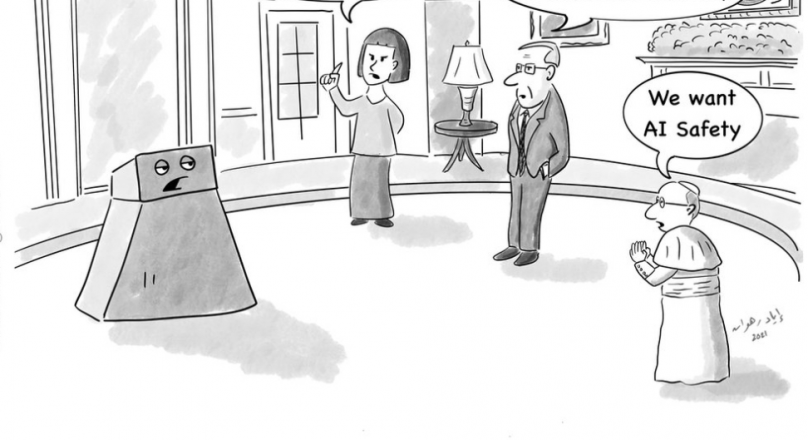
Conclusion:
- Summarize the importance of addressing bias and fairness in AI systems to uphold ethical standards and promote trustworthiness.
- Emphasize the ongoing nature of ethical AI considerations and the need for continuous vigilance, evaluation, and improvement in AI development practices.
- Encourage readers to stay informed, engage in discussions about ethical AI, and advocate for responsible AI implementation in their respective fields.